To achieve sustainability goals, the use of feedstocks like plastics and biomass instead of oil becomes imperative. Additionally, industries are constantly seeking more efficient and sustainable processes, often relying on precise simulations. In the field of heterogeneous catalysis, determining the energy of molecules adsorbed on surfaces plays a crucial role in estimating catalyst performance. Typically, this is achieved through density functional theory (DFT). However, when dealing with large organic molecules found in plastics and biomass, the computational time required becomes enormous, thereby compromising the viability of this approach.
Núria López, Institute of Chemical Research of Catalonia (ICIQ-CERCA), Tarragona, Spain, Alán Aspuru-Guzik, University of Toronto, Vector Institute for Artificial Intelligence, Toronto, and Lebovic Fellow, Canadian Institute for Advanced Research (CIFAR), all Toronto, ON, Canada, and colleagues have developed a model called GAME-Net (Graph-based Adsorption on Metal Energy-neural Network). This model uses a graph neural network (GNN) that rapidly evaluates adsorption energy when molecules attach to surfaces, enabling quick screening of catalytic materials. Notably, this method proves particularly valuable for systems that are too complex to simulate using traditional techniques.
Graph Neural Networks (GNNs) are a specific type of neural network capable of learning the intricate “shape” of data. The algorithm proposed by the researchers employs a straightforward graph representation where nodes correspond to atoms, and edges represent chemical bonds.
The researchers trained GAME-Net using a well-balanced dataset consisting of chemically diverse molecules. The dataset included C1–4 molecules with functional groups like N, O, S, and C6–10 aromatic rings. To begin the process, they created the “functional groups” (FG)-dataset from scratch. This dataset comprised 207 organic molecules adsorbed on 14 different transition metals (Ag, Au, Cd, Co, Cu, Fe, Ir, Ni, Os, Pd, Pt, Rh, Ru, and Zn) on their lowest surface energy facets.
The model achieves a mean absolute error of 0.18 eV on the test set and is six orders of magnitude faster than DFT. When applied to biomass (wood) and plastic molecules containing up to 30 heteroatoms, the model accurately predicts adsorption energies with a mean absolute error of 0.016 eV/atom. Importantly, this technology can be easily run on a laptop, making it accessible to scientists worldwide.
The code for GAME-Net, along with the pre-trained model, has been released as open-source under the MIT license. It is available for download from a repository. GAME-Net is open to further advancements, including the incorporation of specific reaction steps and more complex catalyst materials.
- Fast Evaluation of the Adsorption Energy of Organic Molecules on Metals via Graph Neural Networks.
S. Pablo-García, S. Morandi, R. A. Vargas-Hernández, K. Jorner, Z. Ivkovic, N. López, A. Aspuru-Guzik,
Nature Comp. Sci. 2023.
https://doi.org/10.1038/s43588-023-00437-y
Also of Interest
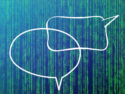
Discussing science communication, AI in chemistry, publication ethics, and the purpose of life with an AI
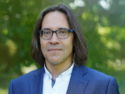
Alexei Lapkin, University of Cambridge, UK, on the state of the art and challenges of transforming chemistry into the digital realm
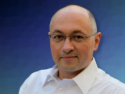
Markus Kraft on the intersection of AI and chemistry, knowledge engineering and machine learning, their applications, challenges and successes, as well as his World Avatar Project